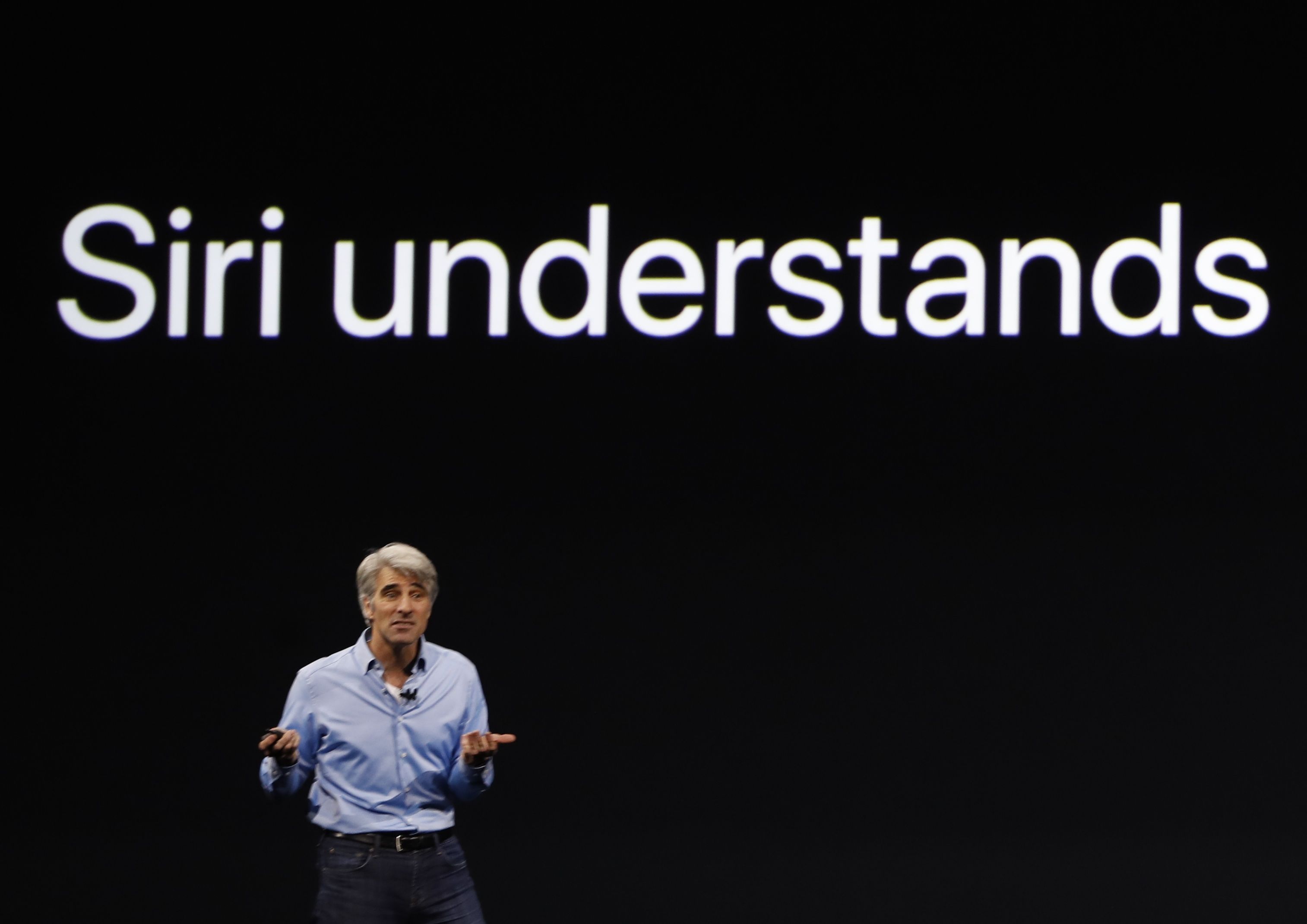
At any given time, most of us are within earshot of a virtual assistant. They’re in our pockets, our houses and our cars.
Whether you’re using Apple‘s Siri to remind you of an appointment, asking Amazon‘s Alexa to play a song for you or consulting the Google Assistant for a local weather report, interacting with these non-human assistants has become normal.
Siri came to the iPhone in 2011, but the underlying technologies are actually older than you might think.
The first machine capable of synthesizing speech was created by Bell Labs 80 years ago in 1939.
In 1952, Bell Labs invented a machine that could understand the spoken numbers 0 through 9. Two years later, in 1954, an IBM machine, in collaboration with linguists at Georgetown, was able to translate 60 Russian sentences into English.
In 1962, IBM created the Shoebox, which could understand 16 spoken words. By 1976, Carnegie Mellon increased that number to over a thousand. And by the mid 1980s, machines could understand tens of thousands of spoken words.
Since then, scientists have started combining these processes with artificial intelligence, a field that itself has been around since the 1950s.
As a result we now have things like Alexa, Siri, the Google Assistant and Microsoft Cortana that are able to understand us when we talk.
Craig Federighi, Apple Senior Vice President Software Engineering
Stephen Lam | Reuters
Different kinds of AI
Artificial intelligence is a big reason computer scientists have been able to make assistants easier to use, but there could be a difference between what you might think of when you hear AI and what it actually means.
“There are two types of AI,” explains Joyce Chai, a professor of computer science and engineering at Michigan State University.
“The strong AI mainly deals with the developing systems that can reason or can think or act like a human. Then the other kind is the weak A.I. which is more focused on specific tasks. And this also includes the virtual assistants. We’re still very far away from strong AI.”
Traditionally, to be able to make a decision about something, a computer needs a set of rules pre-defined by a human. By drawing on machine learning, which is a type of AI, computers are able to infer rules themselves after looking through huge amounts of data.
In this case, they can learn to understand language by looking at how people talk and interact. That requires a lot of data.
Natalie Schluter, an associate professor at the IT University of Copenhagen, explains.
“The main challenge is for these companies is to acquire enough data in enough diverse forms to be able to actually do something for more than one particular person. It might be very interesting in a lab to create a product that can understand you and you alone. But of course there are different dialects, there are different accents, there’s different tones of voice.”
And it’s not just the amount of data—the kind of data matters too. If your training data only comes from white men in San Francisco, you’re going to end up with an AI that can understand a very narrow group of people.
“They have clever people working at Apple and very clever people working at Amazon, ” says Schluter. “But at some point we have to make sure that these people are intervening in the data and making sure that they’re exposed to the right amount of data from a diverse number of people.”
Why Siri is lagging
So why doesn’t Siri always understand what you’re looking for?
In part, it comes down to things that have nothing to do with the science, and everything to do with the reality of how different companies work.
“One of the challenges of Siri is the negative image that they created by over-promising, under-delivering in the early days,” says Keyvan Mohajer, the co-founder and CEO SoundHound, a company that offers a virtual assistant that competes with Siri, along with music-recognition technology and voice tools for other companies to use.
“The other challenge they have is, they haven’t really increased the knowledge base as quickly as you would expect. Amazon went from a handful of skills to hundreds and thousands and tens of thousands. Apple hasn’t really built a developer ecosystem.”
Another possible reason Siri has lagged behind is because of Apple’s strict privacy standards. While many virtual assistants collect as much of your data as possible to train their AI, Apple has been vocal about the importance of minimizing and anonymizing that kind of data collection. While it’s been suggested that this results in a less-useful assistant, Apple strongly disagrees.
“We reject the excuse that getting the most out of technology means trading away your right to privacy,” Apple CEO Tim Cook said at a commencement speech at Duke University in 2018.
Beyond that, Apple is a notoriously secretive company.
“What are people working on, what do they think are really important problems at Apple? We have no idea about that, ” says Schluter.
“Usually at Amazon, at Google, at other companies, Microsoft, we researchers, we all kind of work in the same field and we go to the same conferences. We publish, we collaborate together. Apple is a complete closed book.”
But it does seem like Apple has started to take these things more seriously. Last year it hired John Giannandrea, a renowned computer scientist, away from Google to be its Senior VP of Machine Learning and AI Strategy. And earlier this year it hired Ian Goodfellow, one of Google’s top AI researchers, to be director of machine learning.
John Giannandrea
David Paul Morris | Bloomberg | Getty Images
One study done by Loup Ventures at the end of 2018 showed Siri not yet in the lead, but gaining on its competitors.
Plus just this week at Apple’s Worldwide Developers Conference, it announced updates to Siri Shortcuts, which allows developers greater Siri integration, as well as an update to Siri’s text to speech engine, which now uses a voice generated entirely by software.
But there are still things that Apple could do if it wants to make Siri more impressive.
“The first version of Siri did 12 things,” says Mojaher, “but to be really useful you need to do everything. That’s about coverage and adding more content and having an architecture that allows you to add content and increase to understanding faster than linearly.”
He adds, “I think one of the most promising things that Apple can do is to create a very successful developer community around Siri. I don’t think anybody has done that successfully in the area of voice AI.”